Our latest work just got published in Medical Image Analysis:
Deep learning based Quantitative Susceptibility Mapping (QSM) has shown great potential in recent years, obtaining similar results to established non-learning approaches. Many current deep learning approaches are not data consistent, require in vivo training data or solve the QSM problem in consecutive steps resulting in the propagation of errors. Here we aim to overcome these limitations and developed a framework to solve the QSM processing steps jointly. We developed a new hybrid training data generation method that enables the end-to-end training for solving background field correction and dipole inversion in a data-consistent fashion using a variational network that combines the QSM model term and a learned regularizer. We demonstrate that NeXtQSM overcomes the limitations of previous deep learning methods. NeXtQSM offers a new deep learning based pipeline for computing quantitative susceptibility maps that integrates each processing step into the training and provides results that are robust and fast.
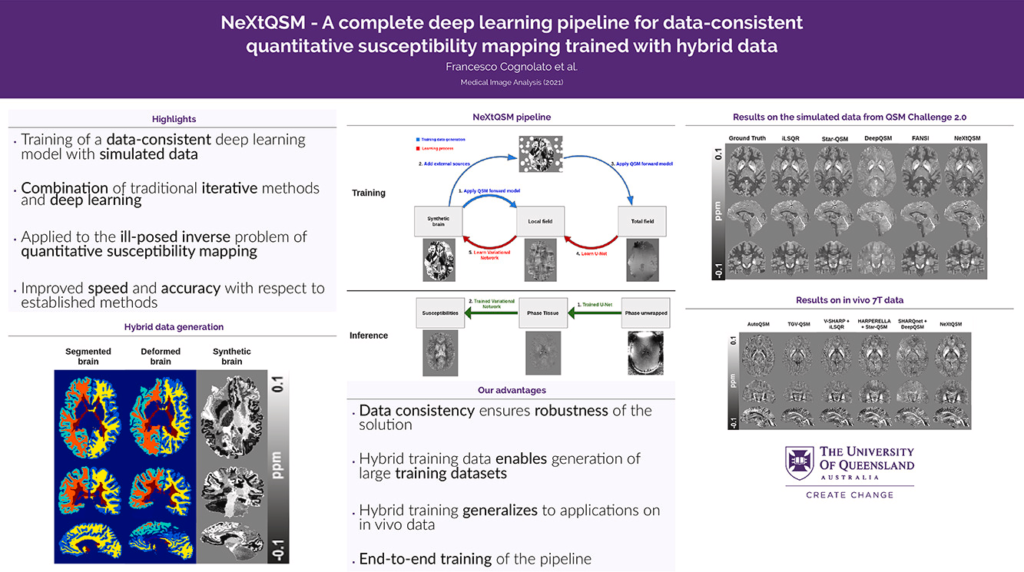
You can access the full article here for free for 50 days: https://authors.elsevier.com/c/1gG5H4rfPmACrG
After that you can find the open-access preprint here: http://arxiv.org/abs/2107.07752
Thank you to all authors for the great work and support and to the reviewers who provided substantial constructive feedback during multiple rounds of revisions.
0 Comments